It takes a team to keep a model healthy in production. ML engineers, data scientists, risk teams, and business users all need to communicate and contribute to ensure that a model is efficient, accurate, fair and drives the bottom line. But not all ML process stakeholders have equal ability when it comes to code. And when MLEs and DSs are swamped, this can result in bottlenecks and waiting time.
This is exactly why we’re releasing no-code model observability integration. With this new capability, any Superwise user can now connect a model and define the model’s schema, and log production data via our UI with just an Excel file.
Iterate faster
Automation and customization are key to achieving scale, but no barriers are key to fast iterations. Sometimes you just want to get started and test something out or need to execute immediately and don’t have time to wait until your entire pipeline is fine-tuned. This is where no-code can step in to release some bottlenecks and allow your teams to move quickly.
Potential use cases
- Proof of concepts
- Businesses with limited developer resources
- Quick time to market applications
- Business users seeking more self-sufficiency
Adding a version and defining schema
All you’ll need is an Excel file of your dataset to upload as a baseline. Then all you need to do is validate the column data types to approve the schema that Superwise has automatically inferred and define the entity roles.
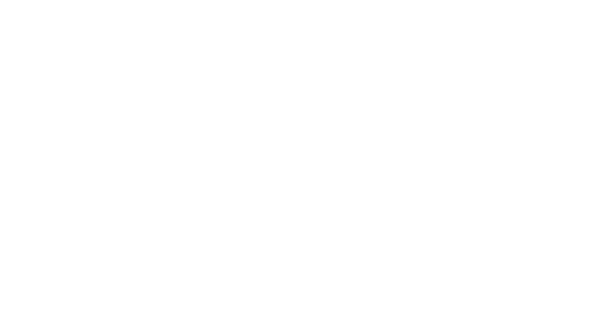
Check out our documentation on uploading a model version here.
Logging production predictions
Logging predictions is even easier, and even with Excel, there are levels of automation available. You can have full flexibility uploading transactions from the UI, or with the help of your development team, you can set up a batch collection that only requires that you dump the files in storage.
Check out our documentation on logging production data here.
Scale is amazing, but the learning curve is sharper without no code. With no code, team members can get started quickly and learn at the same time exactly how to best apply and leverage model observability for their business.
With so many benefits of no-code integration, why not try out connecting your model and data to Superwise yourself? Simply connect a new model and version and send production data with 0 lines of code!
Ready to get started with Superwise?
Head over to the Superwise platform and get started with easy, customizable, scalable, and secure model observability for free with our community edition.
Prefer a demo?
Request a demo and our team will show what Superwise can do for your ML and business.